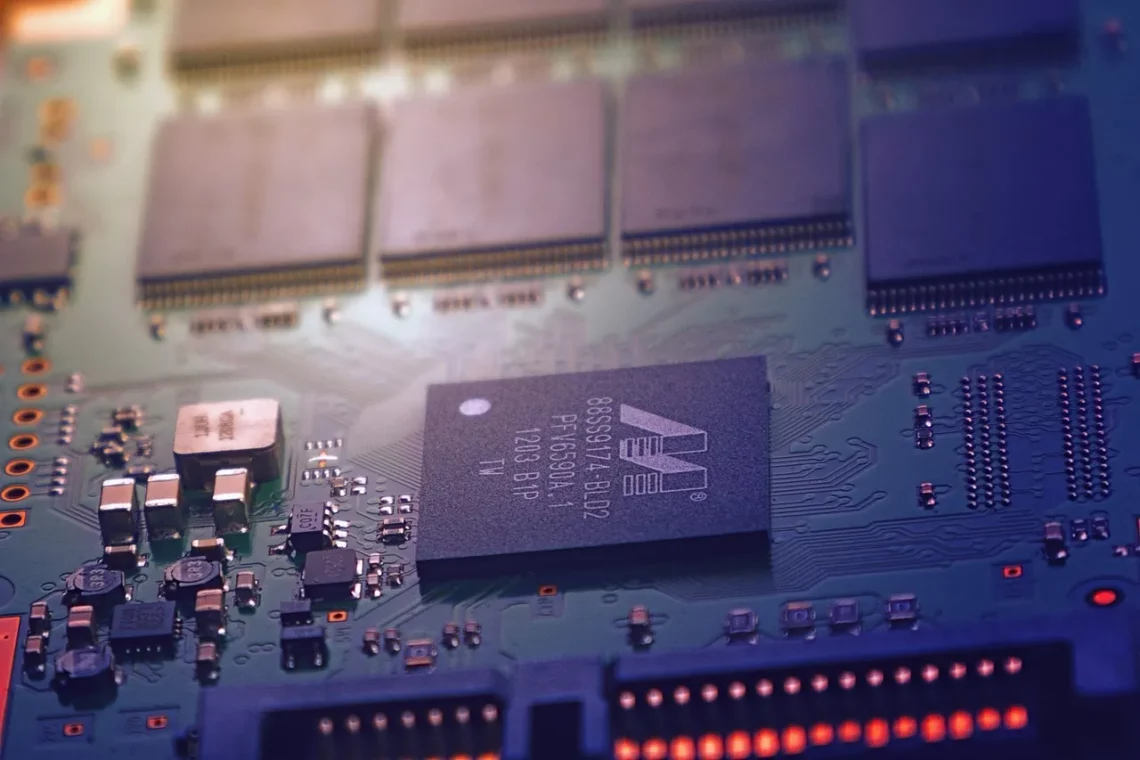
Understanding Mean Cycles: A Guide to Analyzing Data Patterns
Understanding patterns in data is increasingly vital in today’s data-driven world. Whether one is analyzing sales figures, website traffic, or social media engagement, recognizing the underlying trends can significantly impact decision-making processes and strategic planning. The ability to discern cyclical patterns allows businesses and individuals to forecast future occurrences, adjust their approaches, and optimize outcomes.
Mean cycles, which represent the average behavior of variables over a specified period, provide a framework for understanding these patterns. By examining how data points fluctuate around a central mean, analysts can gain insights into both expected and unexpected behaviors. This understanding not only aids in identifying trends but also enhances the reliability of predictions made from the data.
As we delve into the nuances of mean cycles, it becomes clear that they offer a robust tool for interpreting complex datasets. By dissecting the components of these cycles, individuals and organizations can harness their potential to drive informed decisions. In doing so, they not only enhance their operational efficiency but also position themselves to navigate the complexities of the modern data landscape.
Defining Mean Cycles and Their Importance
Mean cycles refer to the average trends observed in a dataset over time, reflecting recurring patterns that can be analyzed for various applications. Understanding these cycles is crucial for different fields, ranging from finance to marketing, as they help stakeholders identify the normal range of variation within their data.
The significance of mean cycles lies in their ability to highlight deviations from typical behavior. When data points consistently fall outside the expected range, it can indicate underlying issues that require attention. For instance, in a retail context, a sudden drop in sales during a traditionally busy season might suggest a shift in consumer behavior or market conditions. By recognizing such deviations, businesses can pivot their strategies to address emerging challenges.
Moreover, mean cycles facilitate better forecasting. By analyzing historical data and identifying cyclical trends, organizations can make educated predictions about future performance. This predictive capability is invaluable in planning inventory, resource allocation, and marketing strategies. Businesses that leverage mean cycles are better equipped to anticipate fluctuations and respond proactively, enhancing overall resilience.
In essence, mean cycles serve as a compass for navigating the complexities of data analysis. They provide a structured approach to understanding patterns, enabling businesses to make informed decisions that drive growth and success. By embracing the insights offered by mean cycles, stakeholders can unlock new opportunities and mitigate potential risks.
Analyzing Mean Cycles: Techniques and Tools
To effectively analyze mean cycles, it’s essential to employ a variety of techniques and tools. The choice of method often depends on the nature of the data and the specific goals of the analysis. Statistical methods such as moving averages, seasonal decomposition, and regression analysis are commonly used to identify and quantify mean cycles.
Moving averages smooth out short-term fluctuations, allowing for a clearer view of long-term trends. By averaging data points over a specified period, analysts can identify the underlying cycle without being distracted by noise. This method is particularly useful when dealing with volatile data, as it provides a more stable view of the central tendency.
Seasonal decomposition is another powerful technique for analyzing mean cycles. This method breaks down a time series into its constituent components: trend, seasonality, and residuals. By isolating these elements, analysts can better understand the cyclical nature of the data and how various factors contribute to overall patterns.
Regression analysis can also play a vital role in understanding mean cycles. By fitting a model to the data, analysts can quantify relationships between variables and predict future outcomes. This approach is particularly useful when examining the impact of external factors on cyclical behavior.
In addition to these statistical techniques, utilizing software tools can enhance the analysis of mean cycles. Programs like R, Python, and specialized data analysis software provide powerful capabilities for visualizing and interpreting data patterns. By leveraging these tools, analysts can gain deeper insights and present their findings more effectively.
Ultimately, the combination of analytical techniques and software tools enables a comprehensive examination of mean cycles. By employing these methods, stakeholders can uncover hidden patterns, enhance their forecasting accuracy, and make more informed decisions based on data-driven insights.
* * *
Take a look around on Temu, which delivers your order to your doorstep very quickly. Click on this link: https://temu.to/m/uu4m9ar76ng and get a coupon package worth $100 on Temu, or enter this coupon code: acj458943 in the Temu app and get 30% off your first order!
* * *
Applications of Mean Cycles in Different Industries
Mean cycles have diverse applications across various industries, showcasing their versatility in data analysis. From finance to healthcare, understanding these cycles can lead to improved decision-making and strategic planning.
In the finance sector, mean cycles play a crucial role in investment analysis. Investors often analyze historical price movements to identify cyclical trends in stock performance. By understanding these patterns, they can make informed decisions regarding when to buy or sell assets. Additionally, mean cycles can help identify periods of volatility, allowing investors to adjust their risk management strategies accordingly.
In marketing, mean cycles are invaluable for analyzing consumer behavior. Businesses can track purchasing patterns over time, identifying seasonal trends and peak periods of demand. This knowledge enables organizations to optimize their marketing campaigns, ensuring they target customers at the right time with the right message. Furthermore, understanding mean cycles can help businesses allocate resources effectively, ensuring they meet demand without overextending themselves.
Healthcare is another field where mean cycles are increasingly relevant. By analyzing patient data over time, healthcare providers can identify trends in patient visits, treatment outcomes, and disease prevalence. This information can inform resource allocation, staffing decisions, and public health initiatives, ultimately leading to improved patient care and outcomes.
Moreover, mean cycles can also aid in supply chain management. Businesses can analyze historical data to anticipate demand fluctuations, ensuring they maintain optimal inventory levels. By understanding the cyclical nature of supply and demand, organizations can reduce costs, minimize waste, and enhance overall efficiency.
In summary, mean cycles have broad applications across various industries, offering insights that drive informed decision-making. By recognizing the cyclical patterns within their data, organizations can optimize their strategies, enhance operational efficiency, and ultimately achieve their goals more effectively.
Challenges in Identifying and Interpreting Mean Cycles
While analyzing mean cycles offers numerous benefits, it also presents several challenges that analysts must navigate. One of the primary hurdles is the presence of noise in the data. Real-world data is often influenced by numerous external factors, leading to fluctuations that can obscure underlying cycles. Distinguishing between genuine cyclical behavior and random variation requires careful statistical analysis and sometimes sophisticated modeling techniques.
Another challenge is the selection of appropriate time frames for analysis. Mean cycles can vary significantly depending on the period under consideration. For instance, a weekly analysis might reveal different patterns than a monthly or yearly examination. Analysts must choose time frames that align with their objectives and accurately reflect the cycles they wish to study.
Data quality is also a critical concern when analyzing mean cycles. Inaccurate or incomplete data can lead to misleading conclusions. It is essential for analysts to ensure that the data they use is reliable and representative of the phenomenon being studied. This often involves rigorous data cleaning and validation processes before analysis can commence.
Additionally, the interpretation of mean cycles can be subjective. Different analysts may draw varying conclusions from the same data set based on their experiences and biases. To mitigate this, it is crucial to adopt a systematic approach to analysis, using established methodologies and seeking peer review when necessary.
Finally, external factors can influence mean cycles in unpredictable ways. Economic shifts, technological advancements, and changes in consumer behavior can all impact the cyclical nature of data. Analysts must remain vigilant and consider these external influences when interpreting their findings.
In conclusion, while identifying and interpreting mean cycles presents challenges, a thorough understanding of these issues can enhance the effectiveness of data analysis. By employing robust methodologies, ensuring data quality, and considering external factors, analysts can navigate these challenges and derive valuable insights from their data.
In summary, mean cycles provide a powerful framework for understanding data patterns across various fields. Through careful analysis and consideration of the tools and techniques available, organizations can unlock the potential of their data, driving informed decisions and strategic success.
This article is for informational purposes only and does not constitute medical advice. For any health-related concerns, please consult a qualified healthcare professional.

